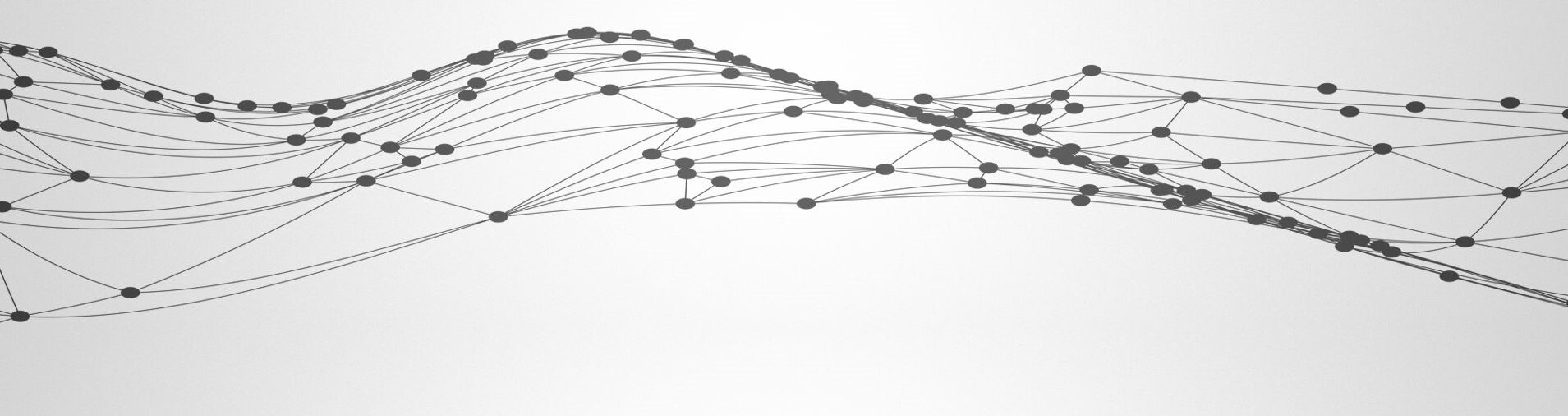
Intelligent Systems Research Group
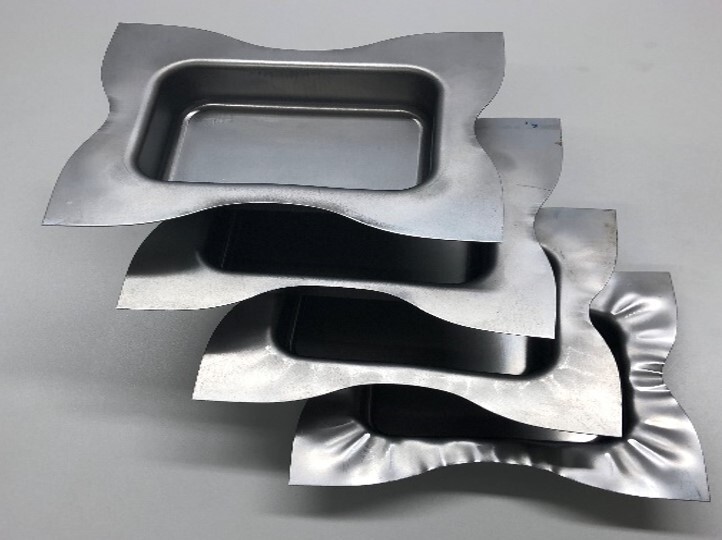
Processes & Materials
The work of the Intelligent Systems Research Group on computational material science, intelligent process control and process monitoring dates back to 2009.
Processes
We have investigated and developed methods that autonomously learn optimal control strategies regarding the process result quality in the context of industrial manufacturing. The basis for such methods can be found in the areas of reinforcement learning and adaptive dynamic programming.
Currently, we are working on reinforcement learning methods to intelligently produce prescribed material structures. The component quality results from the properties of the manufactured component and the achieved material structure at the end of the manufacturing process. We work on methods for optimization of industrial manufacturing process control strategies, which are guided by the current material structure and aim to produce prescribed material structures.
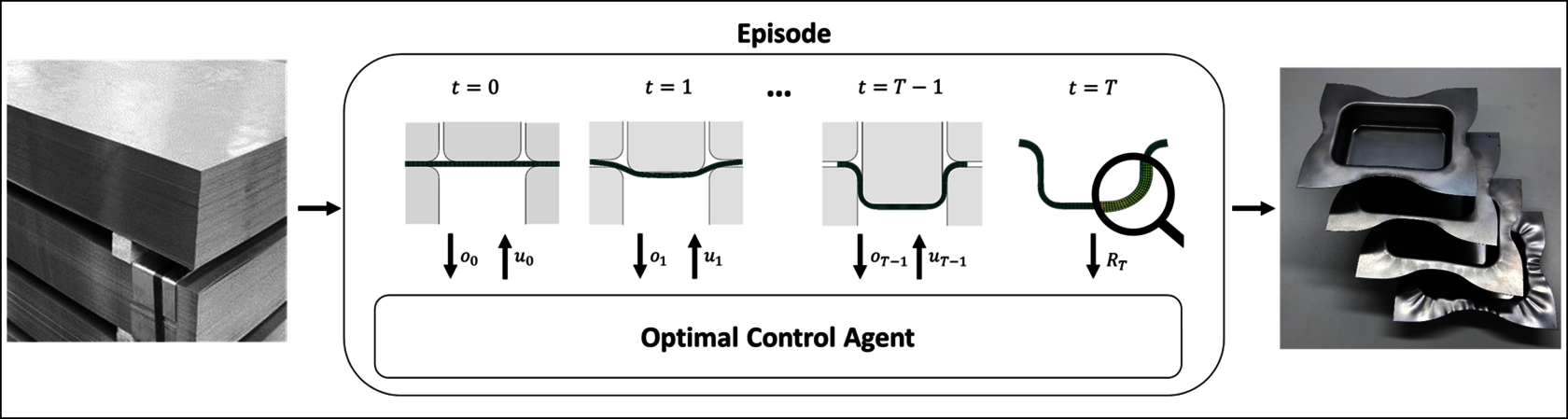
Materials
Workpieces for dedicated purposes must be composed of materials which have certain properties. The latter are determined by the compositional structure of the material. Our research proposes a methodology to automatically find an optimal sequence of processing steps which produce a material structure that bears the desired properties.
The overall task is split in two steps: First find a mapping which delivers a set of structures with given properties and second, find an optimal process path to reach one of these structures with least effort. The first step is achieved by machine learning the generalized mapping of structures to properties in a supervised fashion, and then inverting this relation with methods delivering a set of goal structure solutions. The second step is performed via reinforcement learning of optimal paths by finding the processing sequence which leads to the best reachable goal structure.
Related Publications
Dornheim J., Link N., Gumbsch P.,
Model-Free Adaptive Optimal Control of Episodic Fixed-Horizon Manufacturing Processes using Reinforcement Learning
In Springer International Journal of Control, Automation and Systems, 2020.
Dornheim J., Link N.
Multiobjective Reinforcement Learning for Reconfigurable Adaptive Optimal Control of Manufacturing Processes
Proceedings, 13th IEEE International Symposium on Electronics and Telecommunication ISETC'18, Timisoara, 2018. [pdf]
Fischer S., Hensgen O., Elshaabiny M., Link N.
Generating Low-dimensional Nonlinear Process Representations by Ordered Features
15th IFAC Symposium on Information Control in Manufacturing, 2015.
Senn M., Jöchen K., Phan Van T., Böhlke T., Link N.
In-depth online monitoring of the sheet metal process state derived from multi-scale simulations
The International Journal of Advanced Manufacturing Technology, 2013.
Senn M., Link N.
Monitoring and Control of Process Behaviour
In 22. Workshop Composite Forschung in der Mechanik, 2009.
Partners & Related Projects
The work was carried out on our former research projects, including RTG 1483, ReBorn and I-RAMP³. Currently, we are working in the DFG project MaLISE on a machine learning based solution for the "inverse problem" regarding the three-link chain "process control - microstructure instantiation - material properties". The research was carried out in collaboration with several partners:
- KIT Institute for Applied Materials (IAM)
- KIT Institute of Production Science (wbk)
- KIT Institute of Engineering Mechanics (ITM)
- Fraunhofer Institute for Mechanics of Materials (IWM)
- Constellium
- Robert Bosch GmbH
- Auto-Kabel Management GmbH