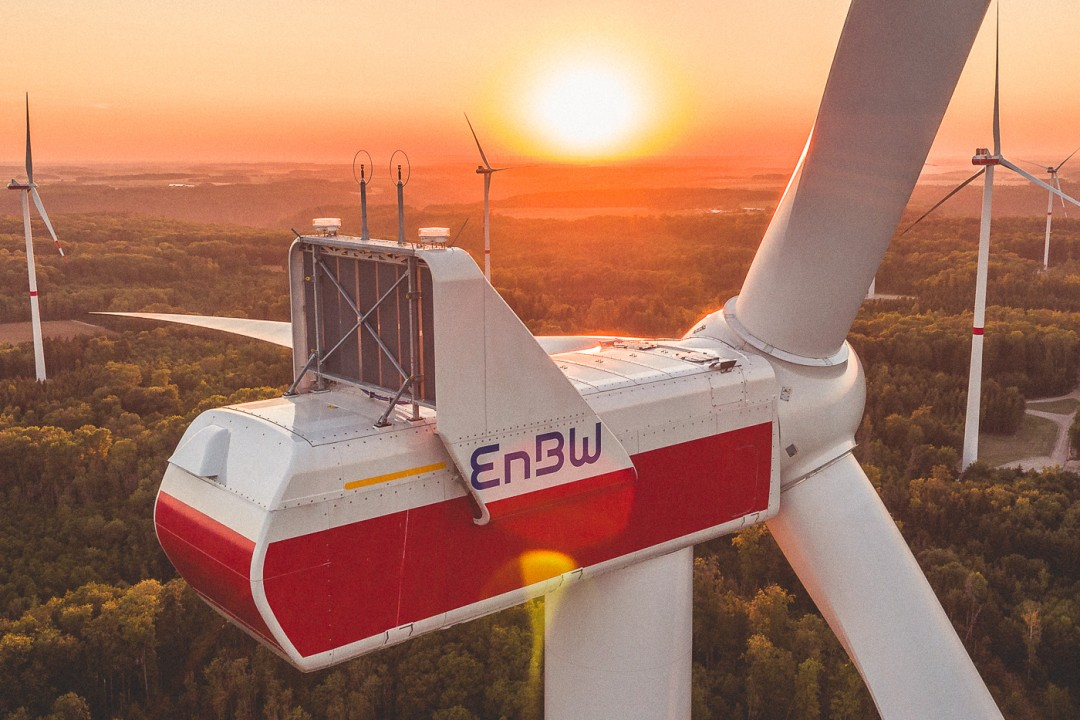
AutoDiagCM
Condition Monitoring 4.0 in wind turbines
Motivation
To achieve the energy policy goals of the German government and the European Union, resources for energy supply need to be efficiently utilized. Condition monitoring plays a crucial role in this regard as it can prevent downtime and consequential damages. Furthermore, precise fault detection enables an increase in energy yield and a transition from reactive maintenance to condition-based maintenance.
The performance of renewable generation systems has been steadily increasing in recent years, resulting in a growing amount of available information. Without machine assistance, it is becoming increasingly challenging to assess the condition of these systems. Each new analysis, monitoring method, or metric increases the need for manual classification by domain experts. As the number of monitored metrics or available signals increases, managing and updating threshold values for anomaly detection becomes more complex and time-consuming. Additionally, the situation is further complicated by different types of information and their temporal resolution (data heterogeneity).
Since damages in wind energy systems can occur rarely and in various forms, self-learning methods require a substantial amount of characteristic damage data. However, such data is often lacking in practice, which limits these methods to providing only general assessments of the system's condition. Specifically, this means that when the model indicates that "the system is anomalous," a manual analysis is still necessary to identify the specific potentially faulty components.
To achieve the goals of expanding renewable energy generation and optimizing maintenance costs, the development of scalable monitoring methods is crucial. The monitoring effort should not increase with the growing number of installations. This requires automated diagnosis of damages and the exchange of insights between systems. Manual diagnostic efforts should focus on rare and challenging damages.
Goals
The Project has five main goals:
- Monitoring the health of wind turbines in its full breadth and depth
- Automation of the diagnostic process
- Continuous model improvement based on historical and previously detected damages
- Transferability of insights and errors to systems of the same type
- Flexibly expandable by integrating additional data sources and analysis methods
By pursuing these goals, a comprehensive and advanced monitoring solution is aimed for, ultimately reducing manual monitoring efforts.
Methods
Smaller models (detectors) continuously monitor individual components and assess their condition. An overarching meta-model is designed to identify problems based on the condition assessments provided by the detectors and assign them to the respective affected components. This approach can potentially achieve high monitoring breadth and depth. By utilizing the condition assessments from the detectors for problem identification and having the detectors only utilize a portion of the sensor signals, the issue of data heterogeneity can be mitigated.
Status
Ongoing project from 01.10.2022 to 30.09.2025
Contact
Prof. Dr. rer. nat. Christine Preisach
E-Mail: christine.preisach @h-ka.de
Tel.: +49 721 925-2599
Partner

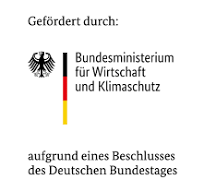
Project Funding
The project is funded by Bundesministerium für Wirtschaft und Klimaschutz.